“AI transformation is no longer an option, it's a necessity.”
Manan Parasher, Founder & CEO
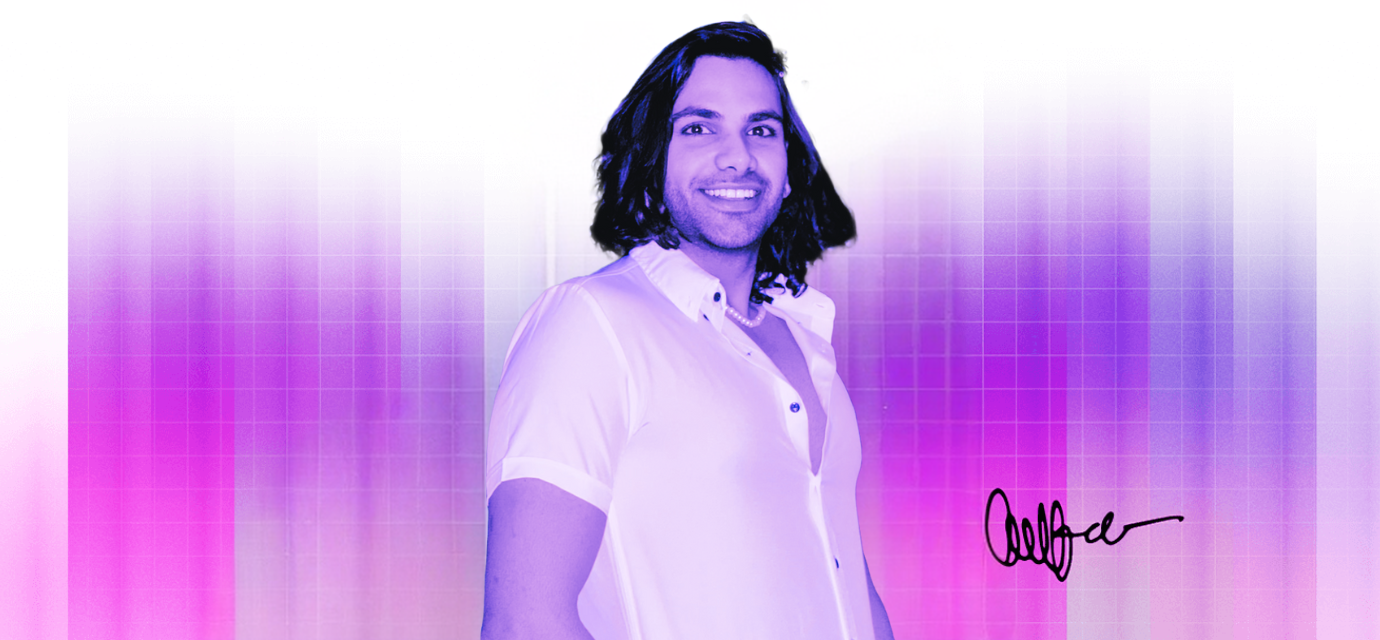
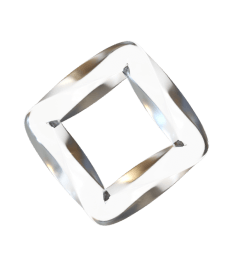
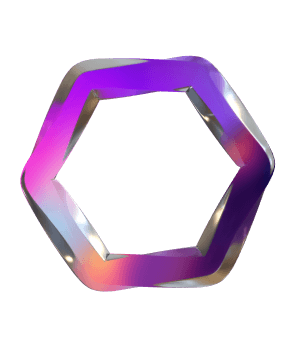
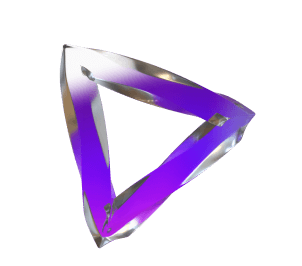
02 — TECHNOLOGYEmbracing Next-Gen AI Technology
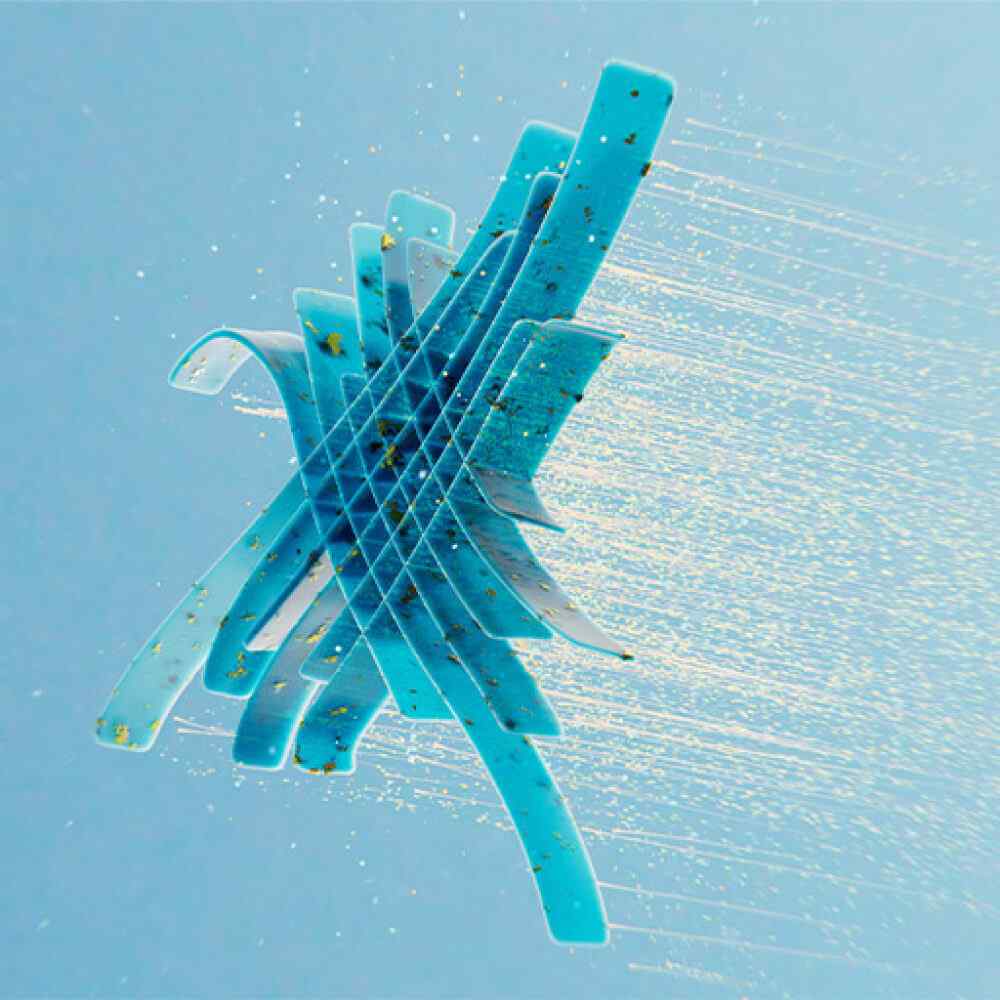
AI MODEL DEVELOPMENT
Crafting Tailored AI Models To Solve Unique Challenges
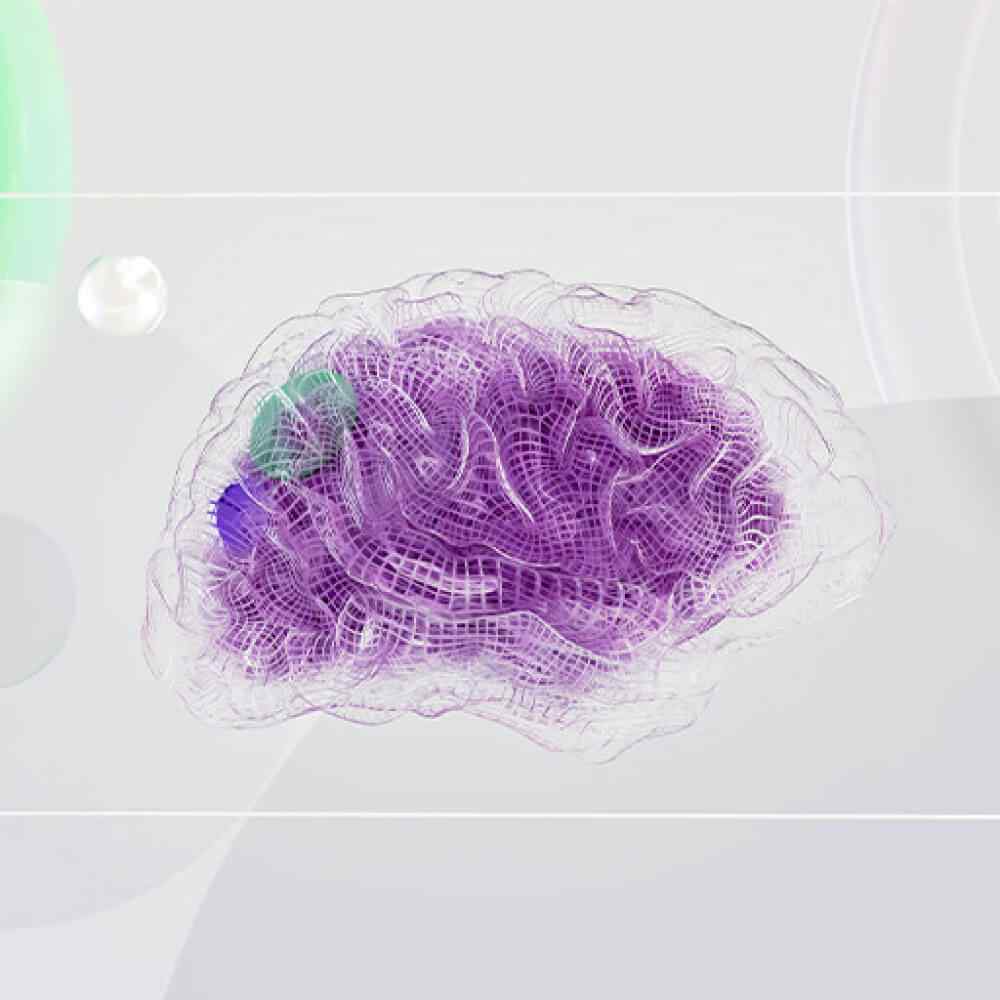
MACHINE LEARNING
Build Personalized Solutions for Goals
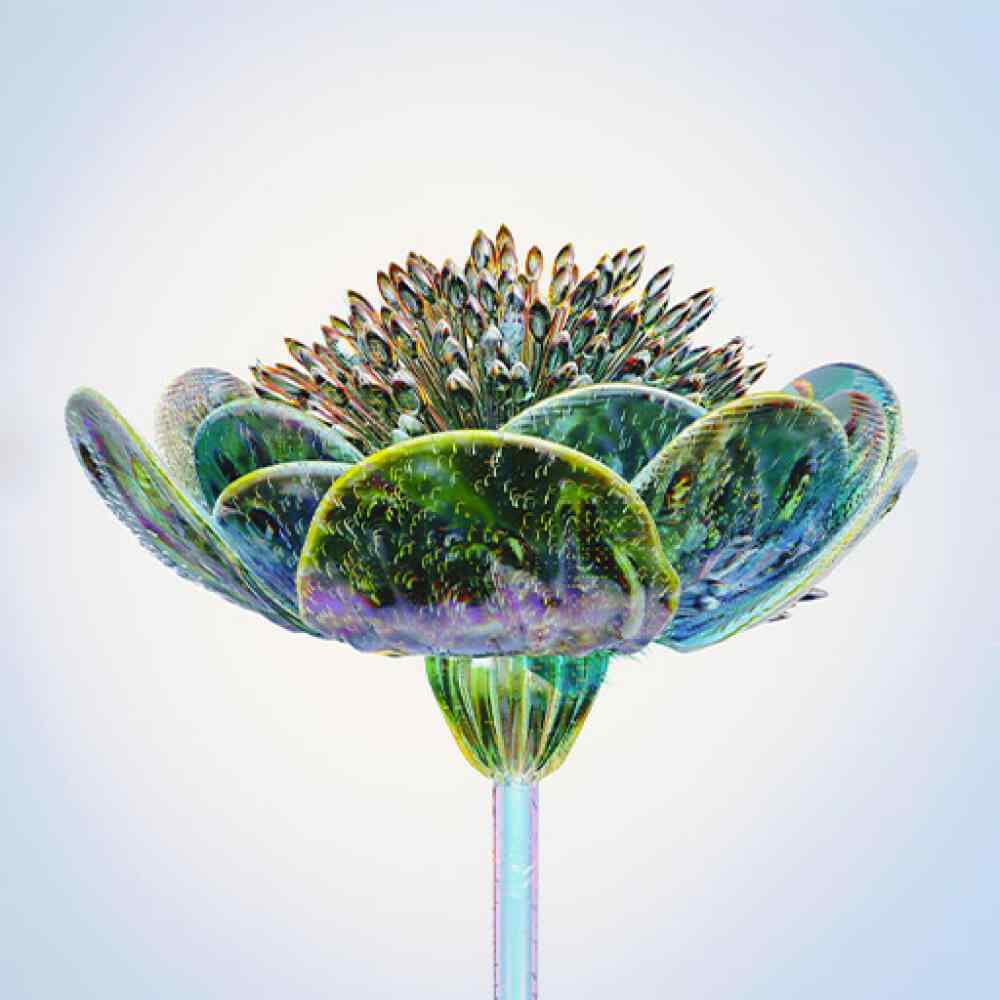
PREDICTIVE MAINTENANCE
AI Solutions That Enabling Proactive Maintenance

03 — CASE STUDIESTransforming Businesses Of All Sizes
From startups to the world’s largest companies

04 — PROCESSAre You Ready To Embrace The Future
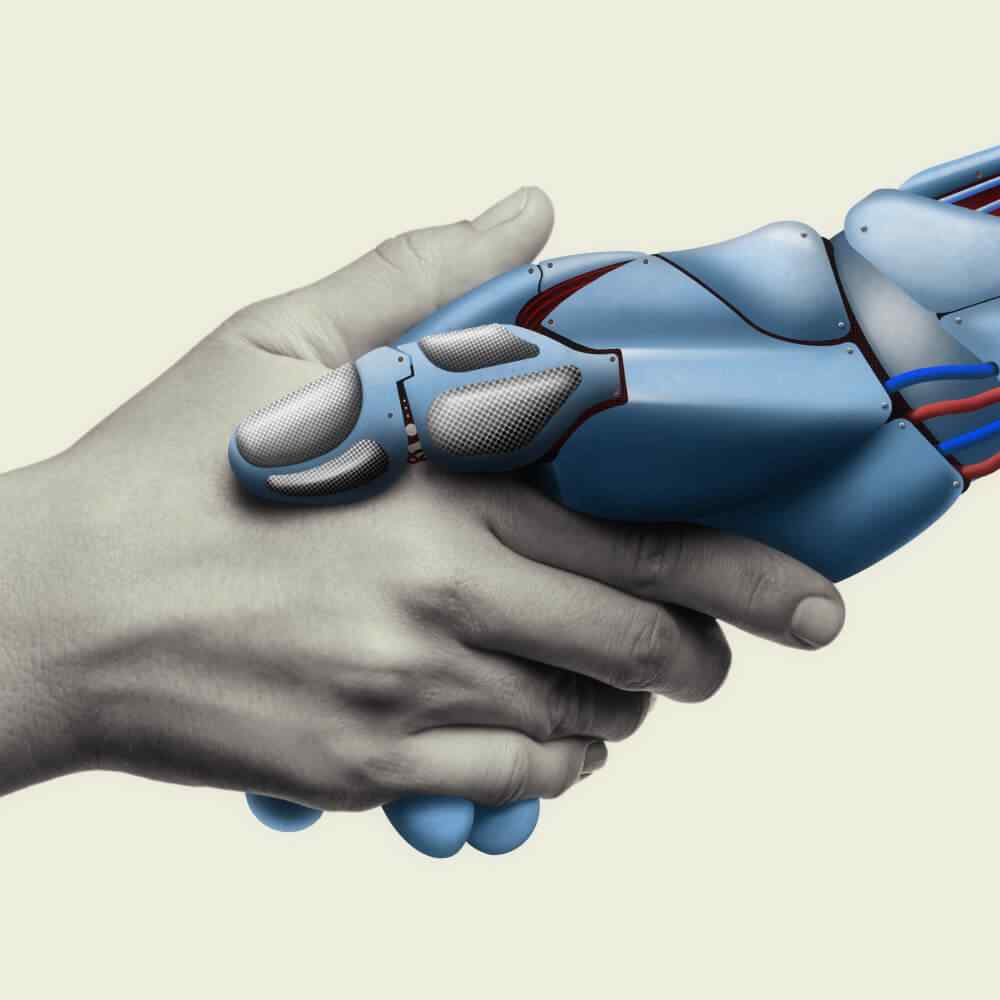
Developing an approach to tackle business problems with AI involves a methodical and structured process. Here’s a comprehensive strategy that outlines each step, from identifying the business problem to integrating and monitoring the AI solution.
1. Identify the business problem
- Define the Problem: Clearly articulate the business issue you’re trying to solve. Is it reducing operational costs, improving customer satisfaction, increasing sales, or something else?
- Set Objectives: Establish specific, measurable goals. What does success look like? Setting clear objectives helps in evaluating the effectiveness of the AI solution later.
- Understand the Impact: Assess how the problem affects the business and its stakeholders. Understanding the problem’s impact can help prioritize efforts and resources.
- Consult Stakeholders: Engage with stakeholders to gather insights and validate the problem’s significance. Stakeholder buy-in is crucial for the project’s success.
2. Gather and analyze relevant data
- Data Collection: Identify and collect relevant data that can help in solving the identified problem. This may include internal data (sales records, customer interactions) and external data (market trends, customer feedback from social media).
- Data Cleaning: Prepare the data for analysis by cleaning it. This involves handling missing values, removing duplicates, and correcting errors.
- Data Exploration: Use statistical analysis and visualization techniques to explore the data, uncover patterns, and gain insights.
- Feasibility Study: Assess the feasibility of using AI to address the problem based on the available data and the preliminary analysis.
3. Develop appropriate AI models
- Select AI Techniques: Choose the AI methodologies and algorithms that are most suited to your problem, such as machine learning, deep learning, natural language processing, or others.
- Prototype Development: Develop a prototype model using the selected AI techniques. This involves selecting features, training the model with a portion of your data, and tuning parameters.
- Model Evaluation: Evaluate the prototype’s performance using appropriate metrics (accuracy, precision, recall, F1 score, etc.). This step may involve comparing different models to find the best performer.
- Iteration: Based on the evaluation, refine and iterate on the model to improve its performance. This may involve additional data collection, feature engineering, or experimenting with different algorithms.
4. Integrate and monitor the solution
- Integration Planning: Plan how the AI solution will be integrated into existing business processes. This might require developing APIs, creating user interfaces, or modifying existing systems.
- Deployment: Deploy the AI model into a production environment where it can start solving the business problem. Ensure that the deployment strategy allows for minimal disruption to existing operations.
- Monitoring and Maintenance: Continuously monitor the solution to ensure it performs as expected. Set up processes to regularly update the model with new data and adjust for any changes in the business environment or objectives.
- Impact Assessment: Measure the solution’s impact against the objectives set in the first step. Collect feedback from stakeholders and use this information to further refine and improve the AI solution.
